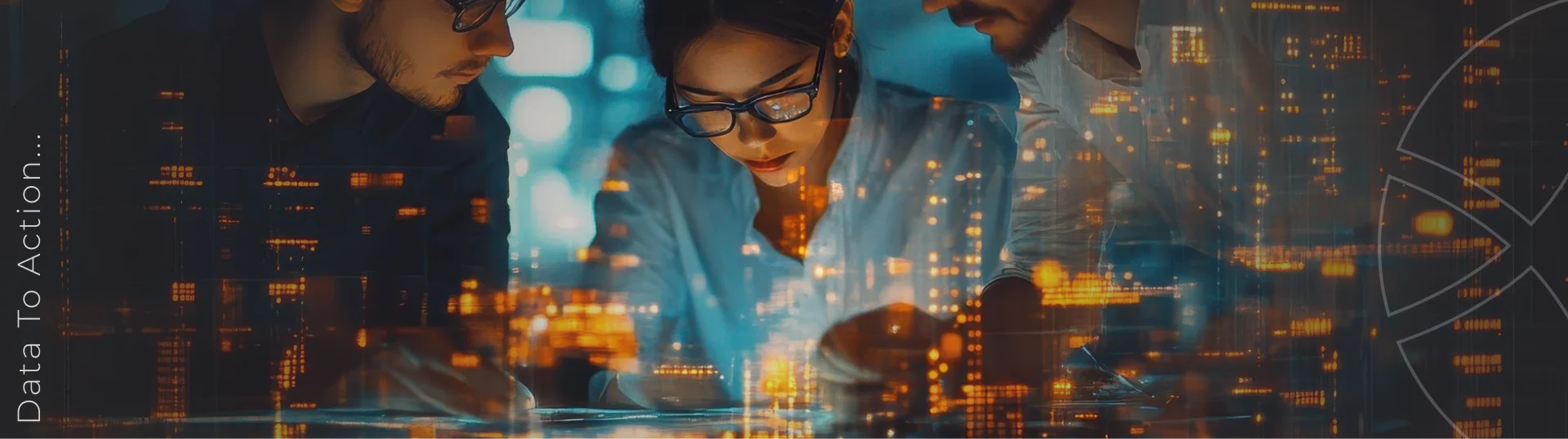
What Is the Ideal Composition of a High-Performance Data Science Team
An end-to-end capability with data analytics prompts companies to organise teams in a certain way. After all, everybody wants an optimised team that can come together, collaborate, and create results.
For the many functions we expect of our data scientists, we expect to hire a few specialists who can focus on core tasks. We hire research scientists, data engineers, casual inference scientists, machine learning engineers, and so on. This team of experts is now expected to zero down on their focus areas, work at their optimal productivity, and turn around with some amazing insights, strategies, and decisions.
Of course, we have a manager to oversee all specialists and become the gel that binds them.
In any other scenario, the goal of a team is to execute a series of actions. They know exactly what they want, and they need people to carry out those functions effectively.
But the aim of data science is not to execute said functions. The primary objective here is to learn and develop new capabilities. If businesses were to follow the existing designs and implement them, they will stay where they are, or only improve by someone else’s standards.
But, we need to work on unique capabilities when it comes to data science. We need exploration and discovery.
So, with data science, you learn as you go. Not before that.
Therefore, when the product is still evolving along with the business, does it make sense to hire a specialist?
Specialisation in Data Science Hinders your Goals
Sounds strange? But here are a few ways how:
- Increases coordination costs – This is the time spent in discussion rooms- going far and wide and deep into problems, defining the approach, setting benchmarks, refining strategies, and bringing all ‘specialists’ on the same page. When you use a data science team organised by function, you need specialists at all steps, with each change and handoff- building up coordination costs. Statistical modeling specialists who experiment with new features will have to coordinate with data engineers who augment datasets at every step of the way. Coordination leads to extra iterations, making it harder and expensive to dissuade exploration- hampering learning.
- Introduces time lapses – While coordination time can be measured by the hours spent in meetings and discussions, the notorious hours spent in defining changes post meetings, resolving dependencies on specialists, and actually implementing changes can be expensive for businesses.
- Narrows context – Who sees the entire picture here? No one. Division of labor by function can limit learning within data science teams by rewarding people for staying in their lanes. Specialists may fail to see outside their confines when they are only supposed to do what they are excellent at. When a research scientist is tasked with optimizing algorithms, they will do that and neglect pursuing everything else, even if that can carry benefits.
The solution?
In order to encourage learning and development within data science teams, the roles need to be made more general and the boundaries overlapping. Organising data scientists so that they can see a complete picture and work in collaboration while still being responsible for a few key functions can increase the overall effectiveness of data analytics efforts.
Employing specialists makes them only fractionally dedicated to holistic business capability. Whereas, with a generalist team, you have people 100 percent devoted to the capability.
With fewer people to loop in, coordination costs reduce. The generalists can fluidly move between functions, offer suggestions, and get things done even if they are out of their area of expertise.
This adds flexibility to the data science team and reduces wait time because all professionals have some idea of each aspect of the process. And, iteration time reduces. One might argue that the generalists would not have the in-depth proficiency of specialists.
But, at this point, we might not be seeking functional excellence or tiny incremental improvements. Instead, we want to discover all possibilities and business capabilities with step-change impact.
With complete business context, each member of a team sees opportunities that a narrowed-down specialist may not.
You might be lured by the idea of improved efficiency in a team of specialists but know that trials and errors have suggested that generalised roles better facilitate growth and learning within organisations.
Build a generalist team when:
- The cost of an error is bearable
- You are not dealing with petabytes or exabytes of data
- Keeping a business capability available and online is mission-critical. More so than improving it
- You have a strong employer reputation to attract the top talent
- You want to promote job satisfaction with autonomy, purpose, and mastery
- You have tools and technologies that can empower generalists to derive value from data
Generalists are not dependent on someone else for the success of a task. They are masters as they know the end-to-end process and the domain. And, they always have a direct connection with the impact they are making within an organisation.
How Can Business Executives Work with Sophisticated Data Science Systems?
Of course, they can. Provided the systems are extremely easy to use, have a short learning curve, and ‘talk’ their language. They should be able to ‘ask’ questions to their data and get answers.
The business people should be able to build models, tune, and deploy them along with the data science team.
With Rubiscape, we aim to provide such a platform. Rubiscape allows non-data scientists make the most of their data. It helps organisations create a data culture without building an army of data scientists. Connect with me if you would like to know more.