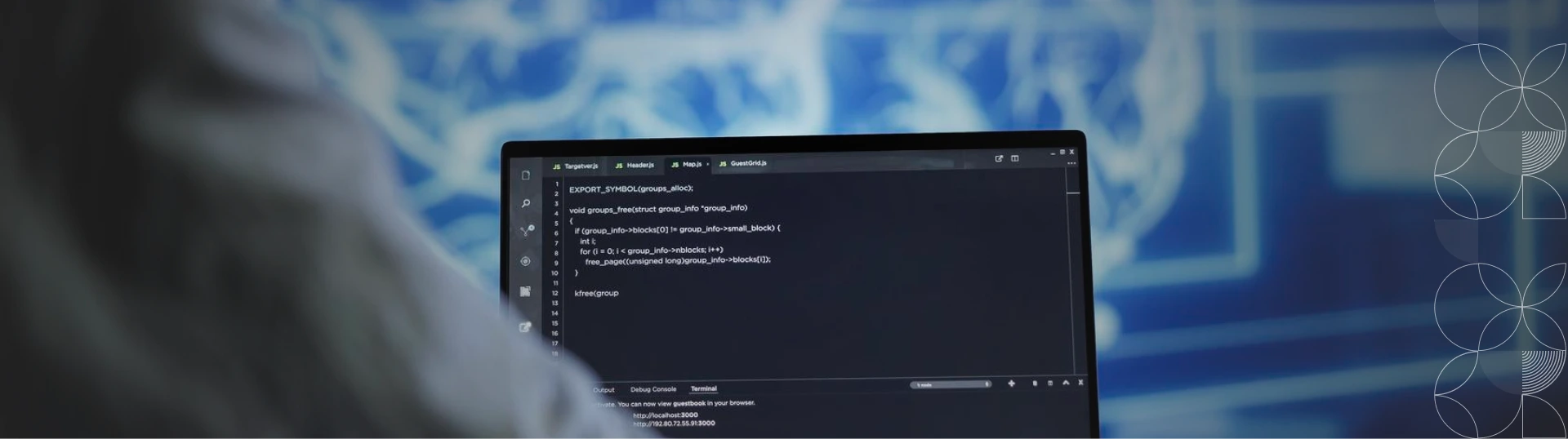
The Techniques, Team, and Tools for Effective Data Science
Have you heard of Airbnb’s Data University? According to Techcrunch, Airbnb has developed its own university-style program with its own courses to train its employees in data science skills. This initiative was taken because, like many other companies, it is struggling to find qualified data scientists. Through this program, it also aims to make its workforce more data literate.
Indisputably, data science is a hot topic amongst CXOs and business leaders. Successful data science projects help companies derive critical data-driven insights, come up with new business models to stay ahead of the competition, and also foster innovation and creativity within the organisation. While the industry leaders understand the potential benefits of data science, running a successful data science project is easier said than done. It is not just about hiring the smartest data scientists – it is a lot more about enabling them through the right data, data accessibility, collaboration, governance, visualisation, tools and techniques, and the right team structure.
Let us take a look at three of the most important aspects – the techniques, roles, and tools for effective data science.
Techniques
“Data scientist is a person who is better at statistics than any programmer and better at programming than any statistician.” — Josh Wills
A good data scientist needs to have a reasonably thorough understanding of statistics, coding, and critical thinking. With increasing diversity and complexity of structured and unstructured data, data scientists need to adopt various techniques and technologies such as AI, machine learning, and NLP to recognise patterns from data, gather insights, and build predictive models from data.
Natural Language Processing
Natural Language Processing (NLP) helps computers understand human language and speech – such as calls, emails, social media content, etc. NLP is used for deep analytics, sentence segmentation, text parsing, machine translation, co-reference resolution, sentiment analysis, semantic text similarity, and automatic text summarisation. NLP is a subset of Artificial Intelligence and is an essential tool for cutting-edge analytics.
Artificial Intelligence
Artificial Intelligence and data science are interlinked. Data scientists today work with a humongous volume of disparate data and artificial intelligence is important for processing this digital data. On the other hand, data scientists develop algorithms and models to process information and build intelligence from that. Automation and intelligence are two key components of AI, and it is about programming computers to build systems to make decisions and do intelligent things.
Machine Learning
Machine learning uses algorithms to search for patterns in data, learn from it and based on that, make decisions and predictions about future trends for that topic. The focus is on creating and enabling algorithms which learn on their own from the provided data, gather insights, and make predictions. Supervised, unsupervised and reinforcement learning are the three most basic models of machine learning.
Team
Data Science is not only about the use of right tech stack – it impacts and is used in a variety of ways within an organisation. Therefore, enterprises need to be extremely careful while building their data science teams. The roles and responsibilities of data science are very diverse, and the skill sets, mindset, and technical knowledge required to perform various data science roles are different. Let’s take a look at various roles in the data science team:
- Data Scientist: This person solves a business problem using data mining and machine learning techniques.
- Data Analyst: This person is responsible for data collection and interpretation.
- Data Architect: This person plays a critical role in data management – to define database architecture, centralise data, and ensure data integrity.
- Data Engineer: This person is an expert in technologies and uses the technical knowledge to implement, test, and maintain the databases and large-scale processing systems.
Building an effective data science team is all about choosing the right people, implementing the right processes, and enabling the team with the right tools. With the gap between the demand and supply of data science skills widening, organisations have started putting their energies on “Citizen Data Scientists” – these are the people who are the domain experts residing within the organisation, are not necessarily data science experts, but using the right tools, are enabled to generate models for predictive analytics. Enterprises are increasingly democratising data science and empowering the “Citizen Data Scientists” with the right tools.
Tools
The success of your data science initiative also depends a lot on the platform you choose for the process. There are quite a few tools for data processing and analysis, but those require technical skills and understanding. You need to build up a team of data scientists. What if you can leverage the benefits of data science without building an army of data scientists? How about using tools which allow you the domain experts within your organisation to easily design workflows, create and reuse predictive models, and run data science – without the need of any technical expertise or data science skills?
Try iDS Cloud, an innovative data science platform which empowers the non-technical business users and analysts to quickly build analytic models and workflows and derive actionable insights from structured and unstructured data.
Built on the Open Source and Hadoop ecosystem, iDS cloud offers pre-built algorithms, a plethora of processes and data sources, support for major BI and data visualisation vendors, and a marketplace for the data science community to enable Simplified Data Science.
Successful data science projects provide actionable insights and not simply correlations. The data science work needs to focus on the things which matter for the business and should offer insights to help the executives take decisions!